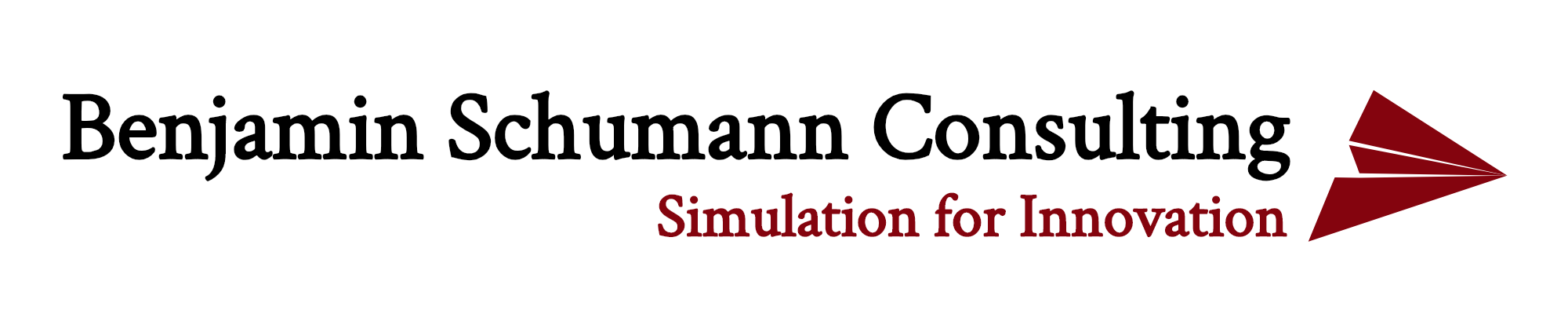
May 9
/
Benjamin Schumann
Is Analytics missing out on simulation?
The other day, I went to an Analytics workshop. I wanted to learn more what is going on at the cutting edge. Big names in the room: Deloitte, SAP, Microsoft, Air Berlin and more. A guy from IBM introduced an asset management tool that eats data for breakfast. I asked him if they use simulation to model assets as part of their tool. In other words, do they actually simulate how things play out in time? His answer stunned me.
"Well, ideally we’d have so much data that we don’t need to simulate anything."
What an interesting view point. But let’s step back for a moment.
What is Analytics all about?
Ok, I am no expert, but in simple terms, Analytics is about improving your insight into a part of reality through data. However, 80% of the work consists of cleaning dirty data and preparing it for some analysis. The rest is crunching those numbers, combining various data sources and applying correlation to achieve insight. The icing on the cake is often some forecasting to arrive at prescriptive Analytics. I don’t mean to diminish this, it is fantastic and super-advanced stuff. However, there is one caveat that I see again and again in data Analytics of real world operations. No matter if it is about logistics, supply chains or asset management. No matter if we talk Uber, British Airways or your electricity company.
The caveat
Let’s assume the best case here: the gentleman from IBM was correct: we do have enough data from our client. We know everything that happened to our client’s operations in the past down to the last screw. A data scientist’s dream, albeit an entirely unrealistic one.
What is wrong with that? In any case, all our data can only ever just show us one version of the past, the one version that actually happened. Below, we see how events unfolded in the past, generating data for us to analyse in the present.
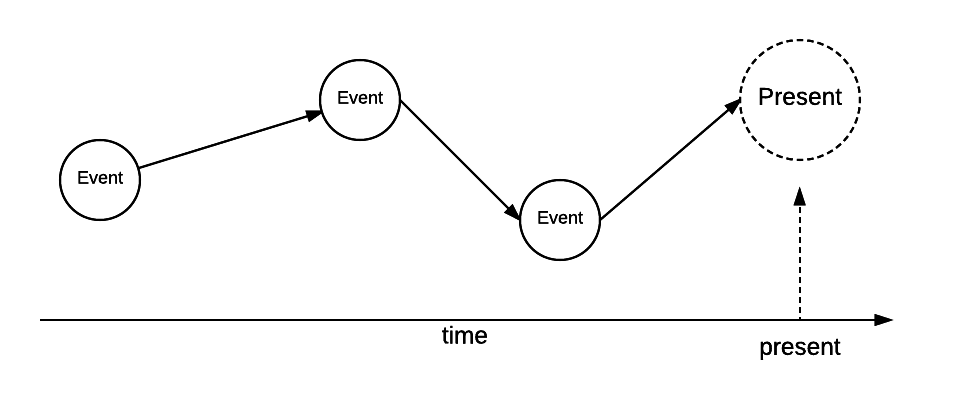
Events in the past create data and we end up with a specific present.
But we live in a complex world. A tiny change to circumstances to an event in the past could have triggered a whole different outcome. In fact, there exist many alternative presents that did not happen but might have happened with a given probability, as below:
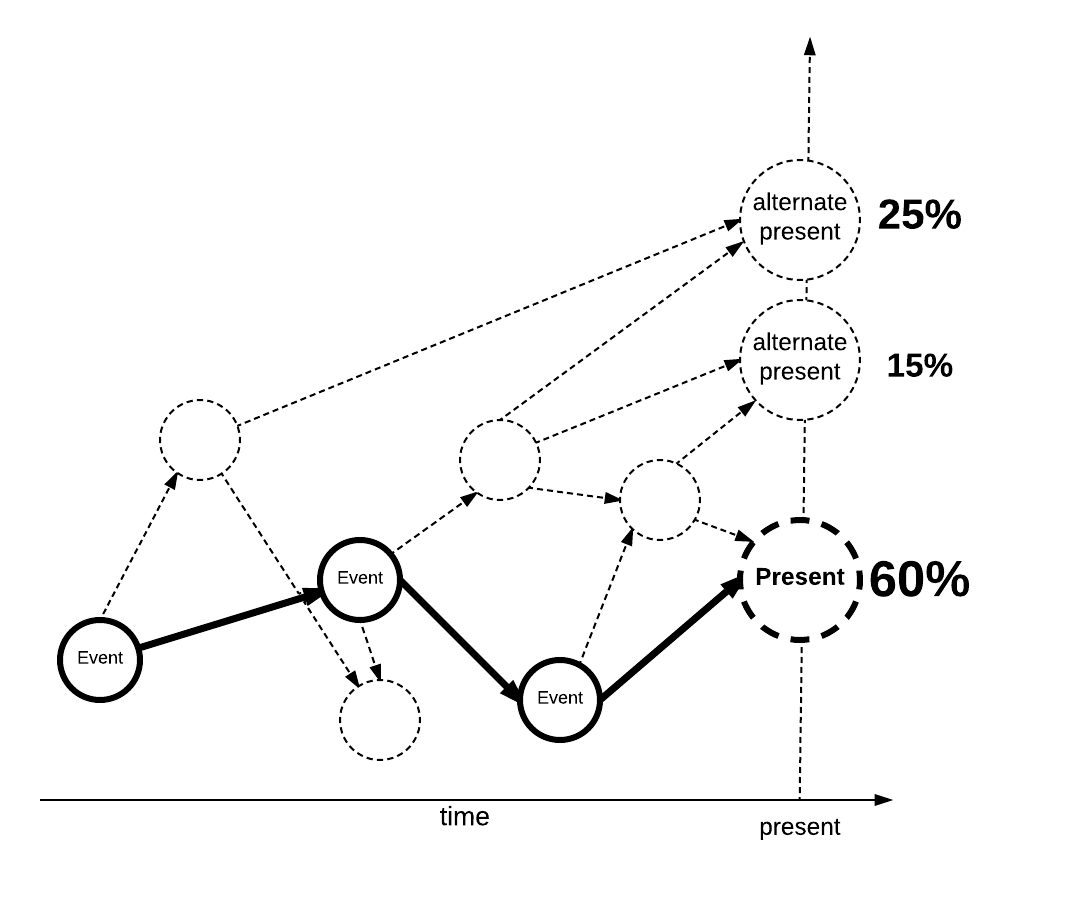
Actually, past events could have created many different presents. Do you take them into account?
I don’t mean to be Stephen Hawking here but this is a simple fact: Data Analytics of past data misses 99.9% of the important data, namely that which did not happen. Worse still, it appears most of predictive/prescriptive Analytics takes our past data and provides forecasts based on past data only. It is like building your sky scraper on a match that should balance the entire building. What if your past data was not actually the most likely past but an outlier? And now you project it into the future, as below:
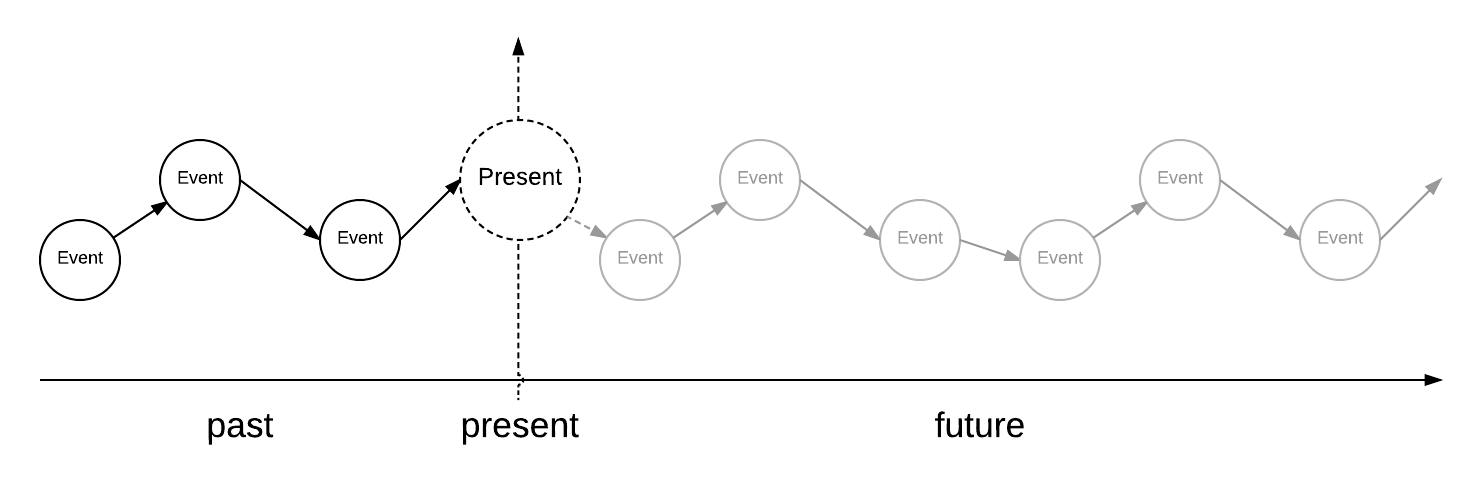
If you only consider the actual past, how good can your predictions really be?
Granted, the forecasting methods are amazing and advance more and more. But they all found on the assumption that our past data is the only possible truth.
How simulation helps
This is where (operations) simulation must join the party. What we do each day is to simulate processes of the real world:
We take past data (like in Analytics) but we only use it to build and verify models that can re-play the past bit by bit. Then, we can replay it with slightly different conditions and random variations, again and again. We can take into account unexpected interactions (think butterfly effect) and add any additional rules from experts. In short, we can build a trustworthy model of your system, including those rough edges that your data might miss.
- We make public transport drive through cities
- We let aircraft engines fly and see how they deteriorate
- We age water pipes and electricity networks
- We deliver toast or the latest smart phone through global supply chains
We take past data (like in Analytics) but we only use it to build and verify models that can re-play the past bit by bit. Then, we can replay it with slightly different conditions and random variations, again and again. We can take into account unexpected interactions (think butterfly effect) and add any additional rules from experts. In short, we can build a trustworthy model of your system, including those rough edges that your data might miss.
Once we trust our simulation, we have a good understanding of the past including what could have happened.
Only then should you start projecting into the future. Only then can you deploy all the fancy concepts of “predictive”, “prescriptive” and so on. Because now, you can base your description of the future on an exhaustive set of the past and take into account alternative futures, see below.
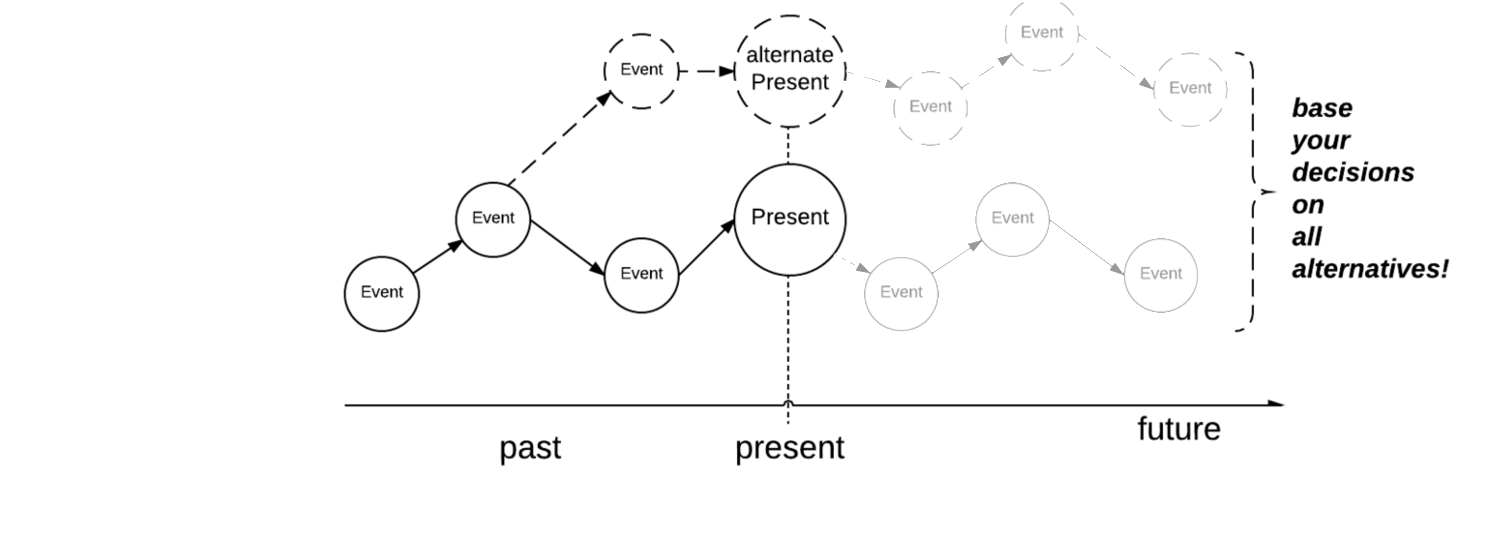
Make sure to use potential pasts to predict the most likely future.
Now let’s take the much more realistic situation where we do not have perfect data from the past. The capabilities of simulation modelling become even more important now. Using heuristics, expert knowledge or simply clever agent rules, our simulations can “fix” missing data (of course we need to make sure it’s done correctly!), see below:
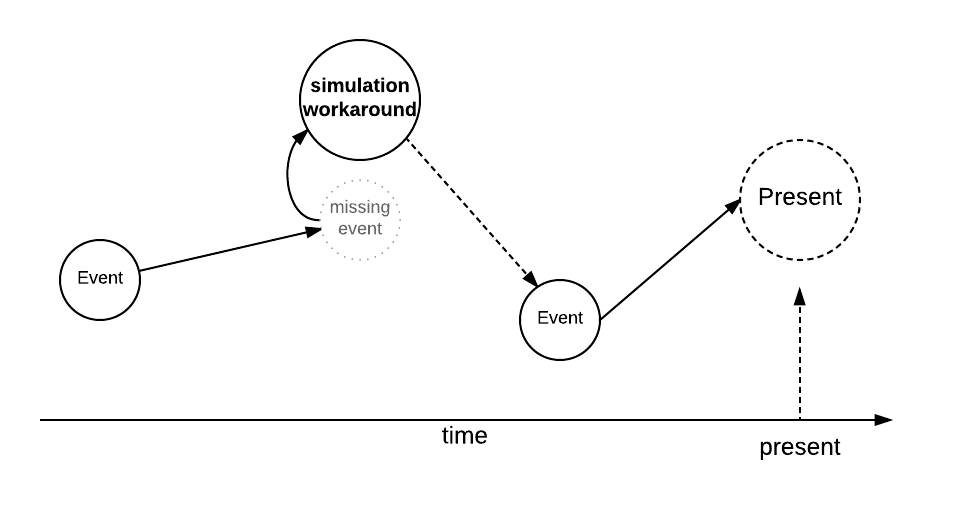
Simulation can even predict missing data from the past.
Disclaimer
I am interested in your thoughts on this, but I should stress one point again: my criticism solely relates to Analytics of real-world operations: asset management, supply chains, logistics. Areas where data from past operations is the central concern. I also realize that sometimes, past data is highly repetitive (daily commutes), allowing to just use that as different versions of the past. However, I would still argue that you do not capture unforeseen behaviour in that data and you cannot employ new policies to see future behaviour.